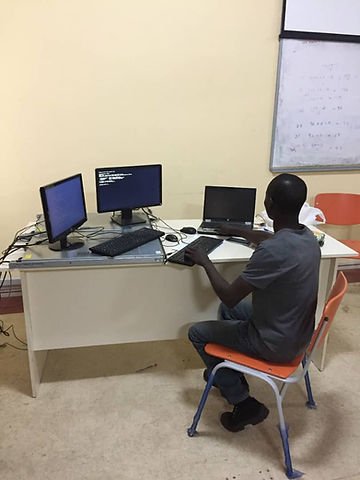
Research Projects

Detection of coronavirus from Chest X-ray
The first and most critical response to curbing the spread of the novel coronavirus disease (COVID-19) is to deploy effective techniques to test potentially infected patients, isolate them and commence immediate treatment. However, a number of test kits currently in use are slow, besides, they are in shortage of supply. In this paper, we present techniques for diagnosing COVID-19 from chest X-ray (CXR). We address problems associated with training deep models with less voluminous datasets and class imbalance as obtained in most available CXR datasets on COVID-19. Further, the models were trained using computational and memory-efficient training techniques that guarantee speedy convergence as well as conserve computational resources, energy, and cost. Despite the availability of scanty datasets, our model achieves high performance and generalization. A Validation accuracy of 96.83%, sensitivity and specificity of 96.26% and 95.54% were obtained, respectively. Lastly, we present a visual interpretation of the model’s output in order to prove that the model can aid radiologists in rapidly screening for the symptoms of COVID-19.
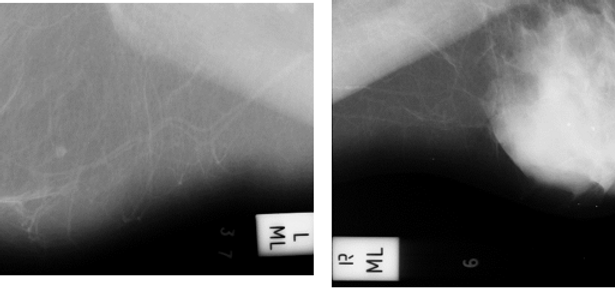
Deep Learning-based Mammogram Classification
using Small Dataset
Breast Cancer is one of the most diagnosed cancer and the leading cause of death among women worldwide, second only to lung cancer. Mammographic screening has been the most successful screening technology capable of detecting up to 90% of all breast cancer even before a lump growth can be felt using breast exam. However, the mammogram is a low-intensity image, and the heterogeneous nature of breast can make healthy breast tissue appears as cancerous, this is most common among women with dense breast (aged 40 – 44). Thus, the sensitivity for early detection of breast cancer from mammogram has been estimated to 85 – 90%. Deep CNN can improve this result; however, to achieve good generalization, it must be trained with high voluminous dataset whereas, mammographic dataset exists in a smaller volume. In this paper, we present a method of training deep CNN with few datasets to achieve high training result and good generalization. An augmentation technique that increases both size and variance of the dataset is presented herewith; the augmented dataset was used to train five state of the art models. Highest training and validation accuracy (99.01% and 99.99% respectively) were achieved with DensNet. Meanwhile, SqueezeNet, a deep CNN model with fewer parameter also shows a promising result, which means soon, we can deploy this model into microcontroller and FPGAs for clinical applications.
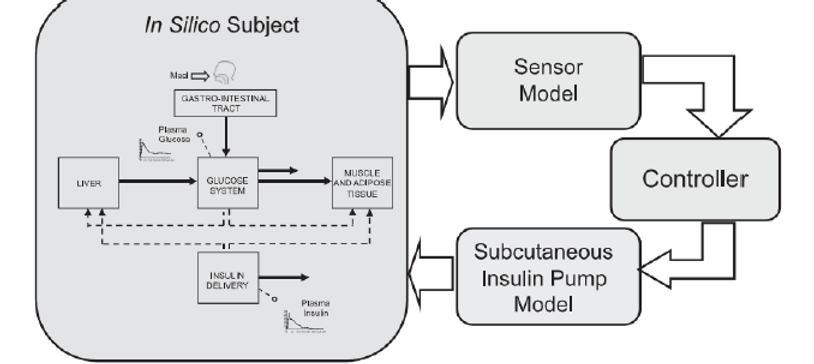
Development of Intelligent Insulin
Injection Controller For Diabetic Patients
Diabetes Mellitus (DM) is a disease of the glucose-insulin regulatory system where the insulin-producing beta-cells
has been damaged thereby producing none to very little insulin leaving the body with no means of regulating glucose. DM has high socioeconomic costs because it needs long term monitoring and individual care to prevent or decrease complications.
Uncontrolled or poorly controlled diabetes leads to evolution or development of microvascular and macrovascular complications. It has been shown that adequate or even tight glycaemic control can prevent or delay complications and finally can reduce these complications. One of these glycaemic control is insulin therapy, meanwhile, non-adherence to the therapy due to its severe pain is prevalent among patients. In this paper, a review of research efforts towards the development of automatic insulin injection from the control engineering perspective is presented. The reviewed techniques are basically the closed-loop approach, which includes PID controllers, Model Predictive Controllers and Adaptive Controller techniques using machine learning approaches.
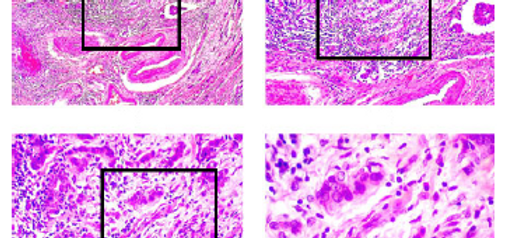
Breast Cancer Histopathology Image Classification with Deep Convolutional Neural Networks
This work addresses the problem of intra-class classification of Breast Histopathology images into Eight (8)classes of either Benign or Malignant Cell. Current manual features extraction and classification is fraught with inaccuracies leading to high rate false negatives with attendant mortality. Deep Convolutional Neural Networks (DCNN) have been shown to be effective in the classification of Images.We adopted a DCNN architecture combined with Ensemble learning method using TensorFlow Framework with Backpropagation training and ReLU activation function to achieve accurate automated classification of these Images. We achieved inter-class classification accuracy of 91.5% withthe BreakHis dataset.
Visit my Research-Gate for more projects: https://www.researchgate.net/profile/Adeyinka_Adedigba or my Google Scholar Page for citation: https://scholar.google.com/citations?user=_jx0tisAAAAJ&hl=en